Written by Tristan Koh and Josh Lee
The regulation of artificial intelligence (“AI”) has been a hot topic in recent years. This may stem from increased societal awareness of: (a) the possibilities that AI may deliver across various domains; and (b) the risks that the implementation of AI may cause (e.g., the risk of bias, discrimination, and the loss of human autonomy). These risks, in particular, have led renowned thought leaders to claims that AI technologies are “vastly more risky than North Korea” and could be the “worst event in the history of our civilisation”.
A key challenge facing any policymaker creating regulations for AI (or, for that matter, any new technology), however, is the epistemic (i.e., knowledge-based) challenge – policymakers must have domain knowledge in order to be able to sufficiently appreciate the scope, size, degree and impact of any regulation, and be able to propose solutions that are effective and pragmatic.[1] In fact, it has been recognised in some governments that subject-matter expertise is lacking when policies or regulations are being crafted.[2] To effectively regulate the development and use of AI, it is clear that policymakers and regulators will need to possess a deep understanding of AI technology and its technical underpinnings.
While a full exposition of AI technology in this short article would not be possible, this article sets out some of the key technical features that policymakers and regulators should consider in the regulation of AI. In particular, this piece focuses on neural networks, a key element in modern AI systems.
Deep learning and neural networks
Neural networks form the architecture of a particularly recent type of AI technology known as “deep learning algorithms”. Deep learning is, generally speaking, “machine learning algorithms that are designed and structured in multiple levels or layers and are inter-connected, resembling a neural network architecture”.[3] These neural networks comprise layers of interconnected nodes and branches (hence the name “neural network”, as the deep learning system mimics – superficially – the structure of biological brain neurons). Each layer of nodes will process a piece of information before the information is then passed to the next layer to process another aspect of it. The output of the system comes when the information has passed through all of the layers, with the results from the various layers adding up to a single output.
Deep learning technologies powered by neural networks have been represented in media as being able to produce spectacular, almost magical, results in tasks previously thought unassailable by machines. AlphaGo Zero, famously known as the system that mastered the strategy game of Go, is one such instance. An important distinction, however, should be drawn: neural networks and deep learning techniques should not be equated with artificial general intelligence (“AGI”). AGI is, put simply, machine intelligence that is able to perform any task that humans are generally able to do (e.g., playing a sport, reading a book, writing a story). This is a holy grail-esque achievement that has yet to be attained and is widely believed to be decades, if ever, away. Thus, while current deep learning systems may be able to perform astonishing feats in specific limited domains, (e.g., playing a game), it is unable to mimic the ability of a three-year old to (for instance) count the number of trees in a park.
The functioning of neural networks and their lack of “explainability”
Neural networks are trained by the forward and backward propagation of values, as illustrated in the diagram below.[4] Information enters the system from the left of the network, and final estimates are returned at the right. To optimise the neural network, the estimates from the right are propagated back towards the left. Depending on how close these estimates are to the actual values of test data, the individual weights of the nodes (i.e., the amount of emphasis placed on the output of each level of nodes) are tuned accordingly. In turn, such tuning improves the accuracy of the neural network’s results. Through repeated iterations, the network is “optimised” by having the most optimal distribution of weights throughout the network that returns estimates closest to the actual values. It is this ability of neural networks to automatically tune their parameters that characterise neural networks as “self-learning”.
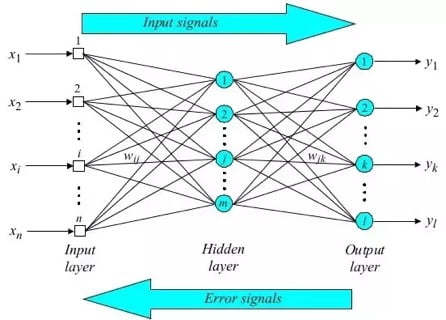
While literature on the optimisation of neural networks is extensive, conventional computing knowledge is still not able to explain how neural networks are able to “self-learn” and provide more accurate results than traditional machine learning techniques (to which end Max Tegmark, a physicist at MIT and author of Life 3.0, has posited that understanding this “self-learning” ability requires not just knowledge of computing, but also physics)[5].
This lack of explainability of neural networks have led to these networks being called “black boxes”, implying that it is difficult to predict results with confidence when parameters of the neural network are changed. This is different from traditional machine learning techniques such as least squares regression (which fits a mathematical function onto data points such that future data can be extrapolated from this function). A simple example of such regression is linear regression, which is described by the mathematical equation “Y = mX +c”. While the mathematics behind other traditional machine learning techniques are more complicated, they are at least comprehensible to humans. For neural networks, this lack of explainability in turn poses legal and ethical risks.
Legal risks and ethical issues raised by neural networks and deep learning systems
The most evident legal issue would be how the lack of explainability affects findings on the remoteness of damage under the tort of negligence. The conventional test of remoteness of damage is the reasonable foreseeability of that type of damage,[6] which has been accepted as the test for remoteness in multiple common law jurisdictions, may not be appropriate when applied to neural networks. For example, it may be challenging to argue that the type of damage resulting from an accident was reasonably foreseeable when the developers might not be able to ascertain with relative certainty how their self-driving car would behave when presented with unconventional scenarios (e.g., how the car would react when detecting defaced road signs).
In fact, it may be argued that the fundamental basis of tort law – fault – could even be challenged when applied to neural networks. It may be challenging to impose tortious liability on the developer of the self-driving software, when the developer may not be able to foresee how its software would react in unconventional scenarios. It may also be challenging to impose tortious liability on the user of the car, when the user of the car is not engaged in the driving task and knows even less about how the self-driving car will function in a specific scenario.
Some experts have argued that a possible solution to this quandary is to impose legal personality (whether full or partial) on the deep learning AI system, and to mandate that the AI systems are to be covered by insurance. This is so that the AI system may be sued, and the resulting damages, if any, can be paid by insurance companies. For instance, this has been proposed for AI systems in the med tech sector.[7] This solution, however, does not appear to be the most principled or intuitive at several levels. First, the proposal essentially shifts the practical burden of liability not to the AI system, but to insurance companies. Second, it is difficult to see how there is a need for the AI system to be imbued with some degree of personhood for incidents arising from their use to be covered by insurance – it would be sufficient to mandate that all incidents arising from AI systems would be covered by insurance without having to impose legal personality on AI systems. Third, based on first principles, it is difficult to see how imposing legal personality on AI systems would reduce their propensity to cause harm. These AI systems would not be able to automatically respond to the imposition of fault and liability the same way that human beings or corporations can – i.e., correcting their behaviour and making sure that the incident does not happen again. The idea of deterrence does not prima facie apply to AI systems without human intervention.
The use of deep learning AI systems would also raise ethical issues. When incidents that affect the public interest occur, regulators are often required to be accountable for such incidents. Regulators would respond, for instance, by setting up inquiry committees to investigate and report their findings. However, if the workings of neural networks remain largely unexplainable, how should regulators be able to explain incidents arising from the use of neural networks and ensure their accountability to society? The potential lack of accountability has the potential to impact the public‘s trust in the adoption of AI.
Building solutions to the problem – explainable AI
Fortunately, there is growing awareness about the need for explainable AI that has developed in response to the relative lack of understanding of deep learning systems. At its heart, explainable AI is a principle to ensure that decisions made by AI systems and the associated data driving those decisions can be explained to end-users and other stakeholders.[8] This translates into design principles, techniques and tools that provide insights into how neural networks make decisions. The Model AI Governance Framework released by Singapore’s Personal Data Protection Commission, for instance, provides guidance on steps that organisations can take to improve their ability to explain how an AI system arrives at a decision. One step suggested is to “incorporate descriptions of the solutions’ design and expected behaviour into their product or service description and system technical specifications documentation to demonstrate accountability to individuals and/or regulators”.[9] In addition, tools such as Google’s TensorFlow Playground allow researchers to adjust parameters in a neural network, providing a simple and graphical way to explain how a neural network arrives at a decision.[10]
In our view, explainable AI forms just one portion of a dual-faceted approach to breaking down the regulation of AI:
- Ascertaining how the AI system functions. This relates to the epistemic issues in relation to AI systems, some of which have been identified above. Explainable AI is part of the larger solution to understanding these epistemic issues, which would like also require an understanding of computer science, physics, mathematics and other domains.
- Ascertaining a desirable outcome for AI systems. This relates to making decisions on what should be done in particular situations where incidents in relation to AI may arise and pose ethical questions. For instance, should we maximise the survivability of passengers or pedestrians in a driverless car? Should we allow governments to implement facial recognition software that is justified by prioritising the safety of its citizens? These are normative questions best answered by legal practitioners, government policy makers and moral philosophers in conjunction with technical expertise from AI engineers.
In this regard, it is heartening to note that the present lacuna in multi-disciplinary research in AI governance is rapidly being filled. One group involved in such research is Oxford University’s Future of Humanity Institute, which “strives to help humanity capture the benefits and mitigate the risks of artificial intelligence”[11]. The multi-disciplinary background of its researchers recognises the fact that AI governance cannot be the endeavour of a single discipline alone. In Singapore, research centres such as the Centre for AI and Data Governance at the Singapore Management University[12] and the recently-established Centre for Technology, Robotics, Artificial Intelligence and the Law and the National University of Singapore have also been set up to look into the complex and multi-faceted domain of AI governance.[13]
Conclusion
Ultimately, modern problems require modern solutions. The discourse of the regulation of tech should not and cannot be constrained to merely the legal field or even the field of legal technology. When encouraging the uptake of technology, we should similarly remember to advocate for the responsible uptake of technology.
Featured Image Credit: Expert Systems
[1] The Policy Framework, https://www.google.com/url?sa=t&rct=j&q=&esrc=s&source=web&cd=1&ved=2ahUKEwiXxtKg0_3lAhW1oekKHQonBv0QFjAAegQIARAC&url=https%3A%2F%2Fapo.org.au%2Fsystem%2Ffiles%2F66816%2Fapo-nid66816-77161.pdf&usg=AOvVaw2l2uq7CEg6JE_Jc7FZhdBu
[2] https://www.instituteforgovernment.org.uk/sites/default/files/publications/Policy%20making%20in%20the%20real%20world.pdf (page 73)
[3] Hannah Yeefen Lim, “Autonomous Vehicles and the Law: Technology, algorithms and ethics”, p 89.
[4] https://aquacoder.com/artificial-neural-network/
[5] Tegmark, Max. 2017. Life 3.0: Being Human in the Age of Artificial Intelligence. Knopf: New York.
[6] Overseas Tankship (UK) Ltd v Morts Dock and Engineering Co Ltd (The Wagon Mound No 1) [1961] AC 388
[7] https://journalofethics.ama-assn.org/sites/journalofethics.ama-assn.org/files/2019-01/hlaw1-1902_1.pdf
[8] Model AI Governance Framework, p 20.
[9] Model AI Governance Framework, p 13.
[10] https://playground.tensorflow.org/#activation=tanh&batchSize=10&dataset=circle®Dataset=regplane&learningRate=0.03®ularizationRate=0&noise=0&networkShape=4,2&seed=0.14888&showTestData=false&discretize=false&percTrainData=50&x=true&y=true&xTimesY=false&xSquared=false&ySquared=false&cosX=false&sinX=false&cosY=false&sinY=false&collectStats=false&problem=classification&initZero=false&hideText=false
[11] https://www.fhi.ox.ac.uk/govai/#home